Rim Ayadi publie un article dans la revue Annals of Operations Research
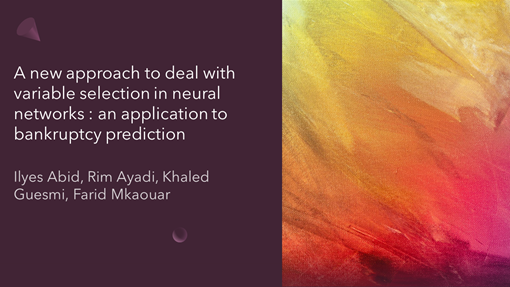
A new approach to deal with variable selection in neural networks: an application to bankruptcy prediction
Abstract
The purpose of the paper is to propose two new procedures that deal with overfitting problem using neural techniques for variable selection and business failure prediction. The first procedure, called HVS-AUC, is based simultaneously on (i) the backward search, (ii) the HVS measure (Heuristic for Variable Selection), and (iii) the AUC criterion (Area Under Curve). The second procedure, called forward-AUC, is based on (i) the forward search and (ii) the AUC criterion. Using a sample of bankrupt and non-bankrupt firms in France, the implementation of the procedures using neural networks shows that the profitability, the repayment capacity, the taxation, and the importance of investment have a strong explanatory power in bankruptcy prediction. These procedures also provide more parsimonious and more efficient models compared to Linear Discriminant Analysis.
Keywords : Bankruptcy prediction · Neural networks · Variable selection · Classification
Référence : Abid, I., Ayadi, R., Guesmi, K. et Mkaouar, F. (2021, online). A new approach to deal with variable selection in neural networks: an application to bankruptcy prediction. Annals of Operations Research. https://doi.org/10.1007/s10479-021-04236-4